
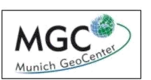
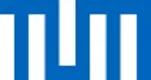
P2: Interpretation of gravity and gravity changes in light of uplift processes
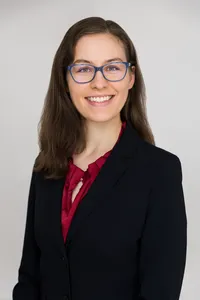
Project leader
Betty Heller-Kaikov
Supervisors
Prof. Roland Pail, Prof. Hans-Peter Bunge
Advisors (int.)
Prof. Martin Werner (TUM)
Advisors (ext.)
Dr. Sia Ghelichkhan (ANU Canberra)
Description
Static and temporal gravity models reflect mass distribution and mass transport processes in the Earth system, and therefore, they are important constraints for geodynamic models (PC IV). Global and regional gravity models will be determined from past and current satellite gravity data and complementary ground data. From combined static gravity field models, which will be computed rigorously (based on the solution of fully occupied normal equation matrices) applying high-performance computing techniques, specific fingerprints of the plate, plume and isostatic mode will be derived. They also represent important constraints for modelling dynamic topography. Geoid trends reflect signatures of all three uplift modes. Measured trends can be used as important constraint for their modelling.
One of the greatest methodological challenges is the separation of very strong near-surface signals (related to processes of land hydrology, cryosphere, atmosphere and ocean) from rather weak mantle signals, which, however, might be distinguished by their space-time behavior. We will investigate and adapt modern algorithms of machine-learning to encounter this challenge. As a starting point we will start with standard models of computer vision and will study their performance in an end-to-end simulation environment. In order to properly consider the time behavior, we will investigate Bayesian structural time series models and adapt them to our purpose. By this, for the first time deep-learning algorithms will be applied to tackle the separation problem.
Motivation
Mantle convection processes contribute to the Earth’s gravity field both due to the direct gravitational effect of the involved density variations, and due to the vertical displacement of the Earth’s surface (dynamic topography). The temporal variations of the Earth’s gravity field are measured globally by dedicated satellite missions such as GRACE and GRACE-FO. While the data accuracy of future satellite gravity missions is expected to be sufficient to detect the mantle-related signals, an additional challenge needs to be solved to extract these signals from the observational data: Since gravity is an integral quantity, only the sum of all mass redistribution processes on Earth can be measured. The aim of the project P2 is to develop algorithms that can separate the individual signals contained in global, temporal gravity data from their measured sum. This is done by exploiting prior knowledge on the typical spatio-temporal behavior of the individual signal components.
Main objectives
- Computation of combined static geoid models incl. realistic error estimates in terms of variance-covariance matrices as constraints for modelling dynamic topography
- Determination of contemporary geoid rates and error estimates from current satellite gravity mission data
- Projection of achievable performance of future double-pair satellite missions, which are expected in the upcoming years
- Separation of near-surface signals from mantle signals by means of pattern recognition algorithms and machine-learning techniques
RTG coupling
Input:
- Satellite and ground gravity data (with special focus on test areas)
- Predicted geoid rates from adjoint geodynamic model (P6) as input for full-fledged end-to-end simulation studies
- Geometric change rates from P1, P3 and P4 as prior and complementary information on uplift processes
- Requirement from P9 regarding uncertainty quantification
Output:
- Combined static gravity field models tailored to test areas (P1, P8, P10), including uncertainty quantification (P9).
- Geoid rates from temporal gravity models (P1, P4, P8, P10) including uncertainty quantification (P9).
- Predicted geoid rates from future gravity field missions (to P1) including uncertainty quantification (P9).
- Spatial patterns related to plume, plate and isostatic mode (all other Ps).
Preliminary results
Using simulated data for the individual signal components in closed-loop simulations, neural network-based algorithms for signal separation are developed and compared to a standard statistical approach based on the Principal Component Analysis (PCA). After first tests using the pix2pix cGAN, a novel algorithm using a multi-channel U-Net architecture is developed and fine-tuned to the task of interest. The algorithms are tested on the task of extracting the GIA signal (S) from a sum of signals (atmosphere&oceans, AO, hydrology, H) and noise (N). Our findings show the applicability of neural networks for solving the signal separation problem, as well as the benefit of exploiting information on the time behavior of the individual signal and noise components, in addition to their spatial behavior. Further challenges still to address are the incorporation of more prior knowledge as additional constraints in the algorithm as well as the application of the developed algorithm to real data.
Papers
- Heller-Kaikov, B., Pail, R. and Werner, M. (2023): Signal Separation in Global, Temporal Gravity Data. In 6th ACM SIGSPATIAL International Workshop on AI for Geographic Knowledge Discovery (GeoAI ’23), November 13, 2023, Hamburg, Germany. ACM, New York, NY, USA, 10 pages. doi.org/10.1145/3615886.3627743
Conference talks
- Heller-Kaikov, B., Pail, R. and Werner, M.: Signal Separation in Global, Temporal Gravity Data. In 6th ACM SIGSPATIAL International Workshop on AI for Geographic Knowledge Discovery (GeoAI ’23), 2023, Hamburg, Germany.
- Heller-Kaikov, B., Pail, R., Werner, M.: Signal separation in global, temporal gravity data using a multi-channelU-Net. European Geosciences Union General Assembly, 2024, Vienna, Austria.
- Heller-Kaikov, B., Pail, R., Werner, M.: Separation of temporal gravity signals using standard statistical and neural network methods. Gravity Geoid and Height Systems 2024 Symposium (GGHS), 2024, Thessaloniki, Greece.
Invited talks
- Heller-Kaikov, B., Pail, R. and Werner, M.: Signal separation in global, temporal gravity data. Oak Ridge National Laboratory, 2024, Oak Ridge, Tennessee, USA.