SparsEO - Sparse Reconstruction and Compressive Sensing for Remote Sensing and Earth Observation
Project Leader
Xiaoxiang Zhu
Contact Person
Xiaoxiang Zhu
Cooperation Partners
German Aerospace Center (DLR)
Chair of Applied Numerical Analysis, TU München
The aim of this project is to develop new theoretical and operational signal processing methods for remote sensing and Earth observation. These methods are designed to address various types of problems that inherently incorporate sparse signals. Particular emphasis is put on (1) the mathematical modelling, (2) the development of efficient algorithms to solve the resulting regularized optimization problems, and (3) the development of overall fast products for operational usage.
Modelling
Image Fusion: Fusion of remote sensing images, which are acquired by complementary sensors of different spatial and spectral resolution, can be used to create imagery of both high spatial and high spectral resolution. In the scope of SparsEO, efficient and robust software products have been developed addressing two of the main image fusion problems in optical remote sensing: SparseFI and J-SparseFI solve the pan-sharpening problem and J-SparseFI-HM solves the hyperspectral-multispectral image fusion problem. All three algorithms build up the fusion product patch-wise as jointly sparse linear combinations of dictionary atoms. One of the most crucial parts in the modelling is the dictionary learning step. Therefore, a dynamic dictionary learning concept has been developed based on patch similarity and probabilistic dissimilarity.
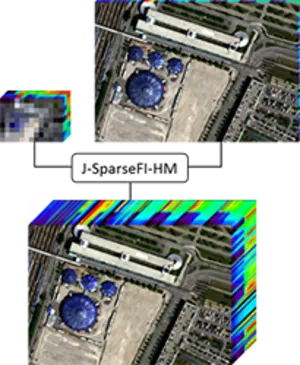
Hyperspectral unmixing: Hyperspectral unmixing aims at identifying materials in hyperspectral pixels. This identification process is realized by comparing the measured pixel’s spectral profile to endmembers (representing pure candidate materials) given in large spectral libraries. In the scope of SparsEO a method has been developed addressing the hyperspectral unmixing problem. It directly exploits the prior knowledge that the number of materials in one pixel is much lower than the number of library entries by means of sparse representation. More specifically, it compares the derivatives of the spectra, which increases the algorithm’s robustness against low signal to noise ratios. Moreover, the high probability of neighboring pixels sharing materials is taken into account modeling the identification process as joint sparse reconstruction problem.
Super-resolution SAR imaging and target identification: The main challenges in identifying targets on oceans and in coastal regions are: 1) The trade-off between coverage and spatial resolution; 2) Side lope with strongly back scattering centers along the coast; 3) Low signal to noise ratio. The aim is to explore the potential of compressive sensing in SAR imaging for target identification. The naturally sparse distribution of objects in coastal regions is exploited by the developed compressive sensing based method to achieve super resolution while suppressing the negative effects that come with side lopes and high noise.
Fast sparse reconstruction solvers
Within the project different sparse reconstruction problems appear that have to be addressed by fast and robust algorithms. In particular, the model error, also referred to as noise on the signal, causes the Noise-Folding Phenomenon and severely affects the efficiency of the algorithms and the accuracy of the computed solutions. To overcome these difficulties, new methods, based on non-convexity, are developed. Such methods depend on certain regularization parameters. One challenge of the project is the tuning of such parameters in order to optimize recovery results for given problem classes. Another thread of development is the parallelization of existing methods to cope with strong demand for fast computational solutions, necessary to process the large amount of data acquired by Earth observation satellites.