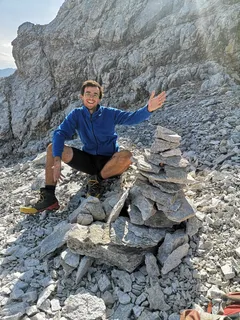
Biography
Michael finished his Bachelor's program Engineering Science in 2018 and graduated in Materials Science and Engineering in 2020 at the Technical University of Munich. Hereby, he focussed on Uncertainty Quantification and Mathematical Modeling - especially Bayesian Inverse Problems. Next to and after his studies, he contributed to projects of the Chairs of Numerical Mathematics, Hydrology and River Basin Management and the Engineering Risk Analysis Group at TUM. Since March 2021 he is a research associate at the Chair of Remote Sensing Technology. The major part of his subject-specific motivation arises from his fascination for outdoor activities.
Research Interests
Michael mainly focusses on methods for the optimization of objective functions in (Bayesian) inverse problems. Buzzwords are Curriculum Learning or Multi-Task-Systems by Multiresolution Analysis.
Both split the original function into sub-functions: the first by weighting the training samples according to it's information content while the error measure stays the same, the second by splitting the original error measure into sub-norms while the used data stays constant. The latter aims at using characteristic properties of basic-functions for the optimization by transforming the original function into a representation by these. They shall ease optimization by incorporating physical properties upon which the basic-functions are based. Further, methods of classic numerics and machine learning are to be combined. In the long run, these basic approaches could be used for Bayesian problems in machine learning or regression in general.
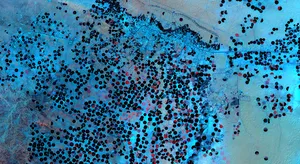
Global Earth Monitor
The Global Earth Monitor (GEM) project is addressing the challenge of continuous monitoring of large areas in a sustainable and cost-effective way. The goal of the project is to establish a new disruptive Earth Observation Data: Exploitation model which will dramatically enhance the utilisation of Copernicus data.