Intelligent Monitoring Algorithms for Automated Data evaluation and Plausibility Checks
Part of the collaborative research project KIMoDIs
Groundwater covers about 70% (regionally up to 100%) of Germany's drinking water demands. Groundwater resources near the surface feed valuable wetland biotopes, as well as the base flow of rivers and streams. As a long-term water reservoir, it also ensures drinking water supply and basic runoff in the event of a temporary lack of precipitation. In many places, however, a shortage of groundwater has become apparent in recent years, which is also perceived by the general public due to increasing media coverage.
Especially in dry summers, the lack of precipitation as a result of short-term climate variability and long-term climate change leads to low groundwater levels, combined with increased pressure of use. In addition to the death of trees, low water levels in rivers and the drying out of groundwater-dependent biotopes, there are also increasingly frequent local bottlenecks in water supply. Since 2018, groundwater levels in Brandenburg and Lower Saxony have fallen to a 30-year minimum at many monitoring sites. In many places, low groundwater levels are associated with salinisation due to the rise of saline deep waters or seawater intrusion near the coast. Studies based on climate projections show that the trend towards drier and warmer summer half-years will continue until 2100, with a presumably increasing demand for water, especially for agricultural irrigation. So far, there is a lack of intelligent tools for forecasting and early warning of extreme groundwater levels with corresponding options for counteracting them, e.g. through adapted management of resource use. Long-term developments, which would have to be used as a basis e.g. in the licensing practice for water withdrawals, have also not been considered so far. The reason for this is the complex interrelationships and numerous, largely time-variable influencing factors such as climate, land use, hydrogeological factors, interaction with surface waters as well as water withdrawals (e.g. irrigation).
Although the movement of groundwater largely follows known physical processes, modelling with analytical or numerical models at the state or national level at a target-oriented spatial resolution is not yet possible. At best, smaller models are operated in the catchment area of individual water suppliers. AI methods, especially the neural networks or deep learning methods belonging to the field of machine learning, which are often used for pattern recognition, offer significant added value here compared to the established methods. As data-based models, they are able to extract complex relationships from existing data and transfer them both spatially and temporally. They appear to be particularly well-suited for modelling groundwater levels and salinisation, since a very good database with high spatial density, temporal coverage and frequency is available for the parameters under consideration, namely groundwater level and electrical conductivity. Applied, groundwater-related AI applications are rare so far, and the transfer of innovative AI solution approaches in the groundwater sector from university and institutional research to practice is to be advanced with this project.
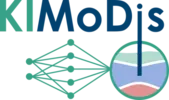
The aim of the KIMoDIs project is the continuous, early, and reliable assessment of groundwater levels and their quality. For this reason, a particular focus of this subproject is on the automated and exhaustive evaluation of all measurement and observation data considered, especially against the background of the strongly pronounced heterogeneity and multimodality of these data sets. In addition, a continuous evaluation of the gained knowledge with regard to its quality, reliability, and validity will be necessary.
Our subproject aims to develop innovative data-driven and hybrid modelling approaches to derive groundwater levels and salinities from Earth observation data or to predict them in the form of forecasts. For this purpose, circulation models are also to be created in order to identify and quantify transport processes. By including dense time series of such observations, the seasonal context, in particular, is to be taken into account, whereas the now very high spatial resolution incorporates the regional context in a value-creating way.